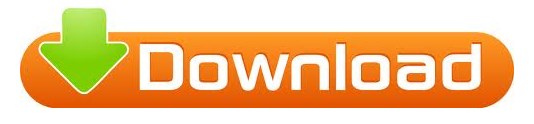
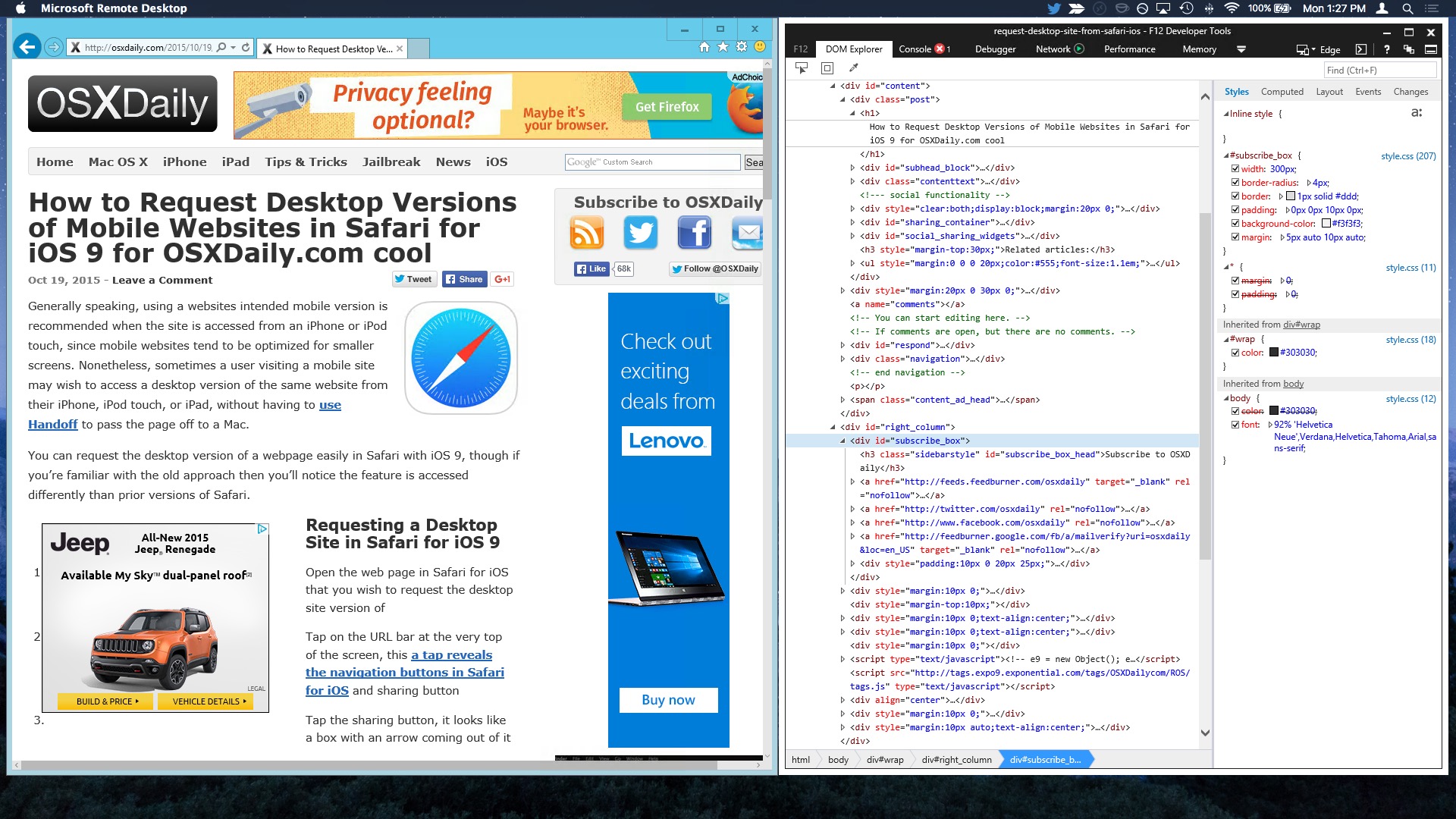
- #MULTIPLE LINEAR REGRESSION EXCEL FOR MAC 2011 HOW TO#
- #MULTIPLE LINEAR REGRESSION EXCEL FOR MAC 2011 FULL#
- #MULTIPLE LINEAR REGRESSION EXCEL FOR MAC 2011 TRIAL#
This example shows how to run regression in Excel by using a special tool included with the Analysis ToolPak add-in. How to do linear regression in Excel with Analysis ToolPak
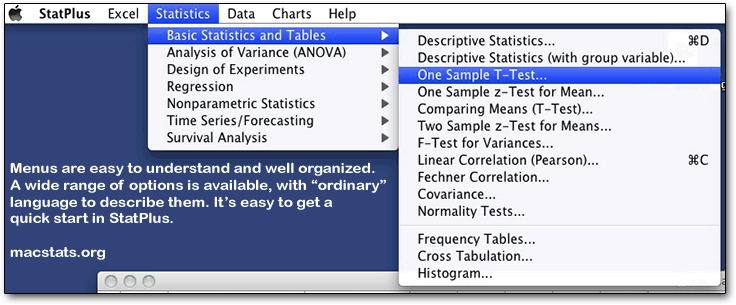
Plot this information on a chart, and the regression line will demonstrate the relationship between the independent variable (rainfall) and dependent variable (umbrella sales): The focus of this tutorial will be on a simple linear regression.Īs an example, let's take sales numbers for umbrellas for the last 24 months and find out the average monthly rainfall for the same period. If the dependent variable is modeled as a non-linear function because the data relationships do not follow a straight line, use nonlinear regression instead. If you use two or more explanatory variables to predict the dependent variable, you deal with multiple linear regression. Simple linear regression models the relationship between a dependent variable and one independent variables using a linear function. In statistics, they differentiate between a simple and multiple linear regression. The goal of a model is to get the smallest possible sum of squares and draw a line that comes closest to the data. Technically, a regression analysis model is based on the sum of squares, which is a mathematical way to find the dispersion of data points. Regression analysis helps you understand how the dependent variable changes when one of the independent variables varies and allows to mathematically determine which of those variables really has an impact. Independent variables (aka explanatory variables, or predictors) are the factors that might influence the dependent variable. In statistical modeling, regression analysis is used to estimate the relationships between two or more variables:ĭependent variable (aka criterion variable) is the main factor you are trying to understand and predict. Regression analysis in Excel - the basics Regression analysis in Excel with formulas.Linear regression in Excel with Analysis ToolPak.It will give you an answer to this and many more questions: Which factors matter and which can be ignored? How closely are these factors related to each other? And how certain can you be about the predictions? But how do you know which ones are really important? Run regression analysis in Excel. You have discovered dozens, perhaps even hundreds, of factors that can possibly affect the numbers. Imagine this: you are provided with a whole lot of different data and are asked to predict next year's sales numbers for your company. Your privacy is assured.The tutorial explains the basics of regression analysis and shows a few different ways to do linear regression in Excel.
#MULTIPLE LINEAR REGRESSION EXCEL FOR MAC 2011 TRIAL#
* The trial lets you try all the features of Analyse-it (including multiple linear regression add-in) with no commitment to buy. There's no new interface to learn, no locked-in file formats, and you can easilyĮxchange your data and analyses with colleagues that have Excel. Save Fitted Y, Residuals, Standardized Residual, Studentized Residual, Leverage, and Cook's Influence back to the dataset for further analysis.Įasily predict future observations using the model.Ĭonduct all your statistical analysis without leaving Microsoft Excel. Identify outliers and cases with high influence with the Outliers & Influence plot using Cook's D and Studentized Residuals. Easily examine the effect of each term using leverage (partial residual) plots and sequential (type I SS) and partial (type III SS) hypothesis tests.ĭiagnose model fit with raw and standardized residual plots, sequence, lag and residual distribution plots.
#MULTIPLE LINEAR REGRESSION EXCEL FOR MAC 2011 FULL#
Or build complex multiple regression models with simple and polynomial terms, factors, and crossed factors, all the way up to full factorial models, ANOVA, ANCOVA, all with automatic dummy variables.įit statistics include parameter estimates, R2, R2 adjusted, AIC, BIC, VIF, Correlation/Covariance of estimates matrices, model equation. Simple regression & Advanced regression modelsįit simple regression models with linear, logistic, probit, polynomial, logarithmic, exponential, and power fits.
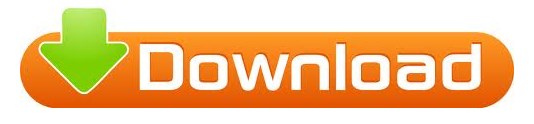